Google Cloud's Future of AI: Perspectives for Startups, featuring AssemblyAI
As part of Google Cloud's AI trends report, AssemblyAI founder and CEO Dylan Fox shares his perspective on last mile issues for AI in startups.



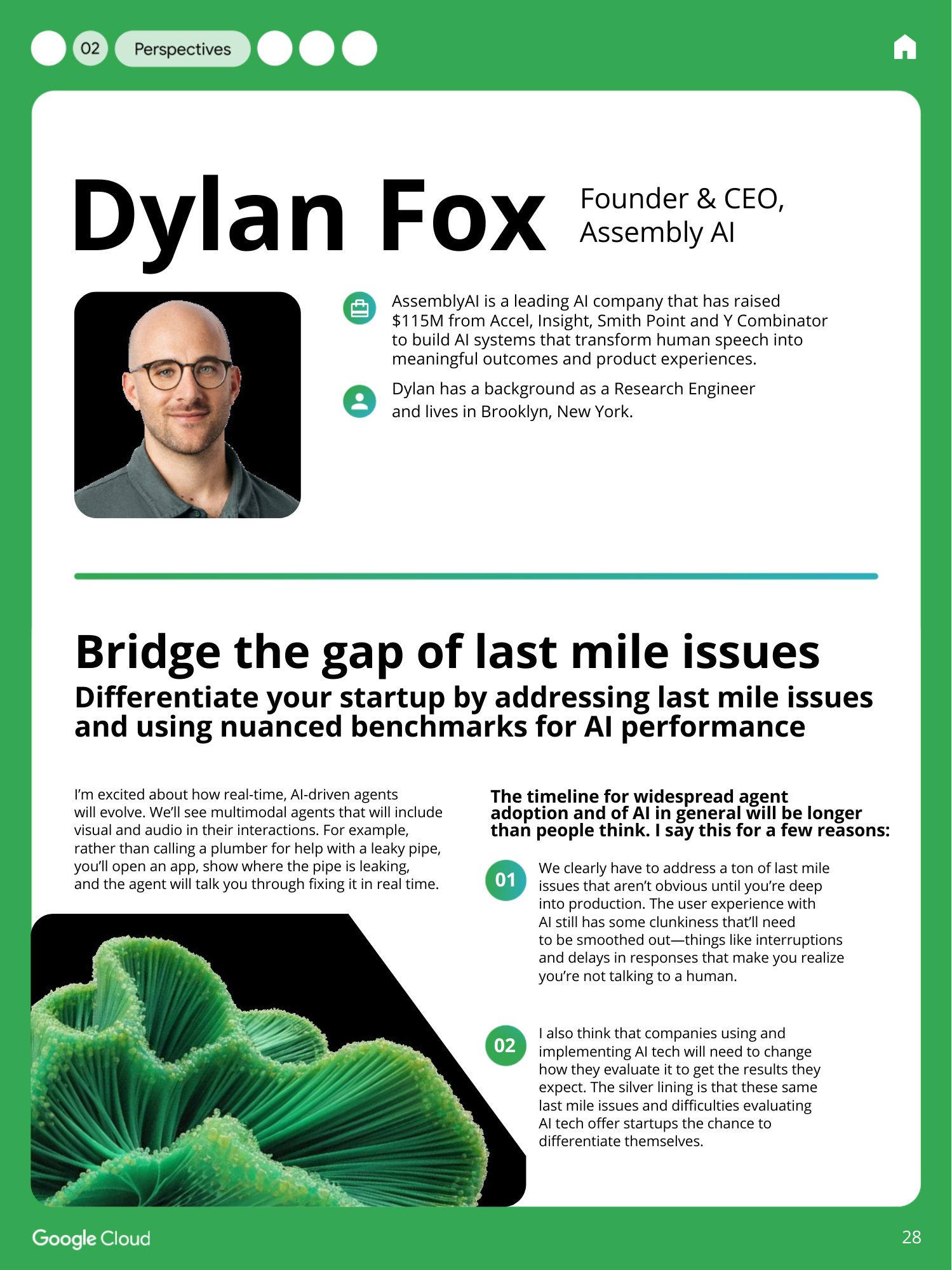
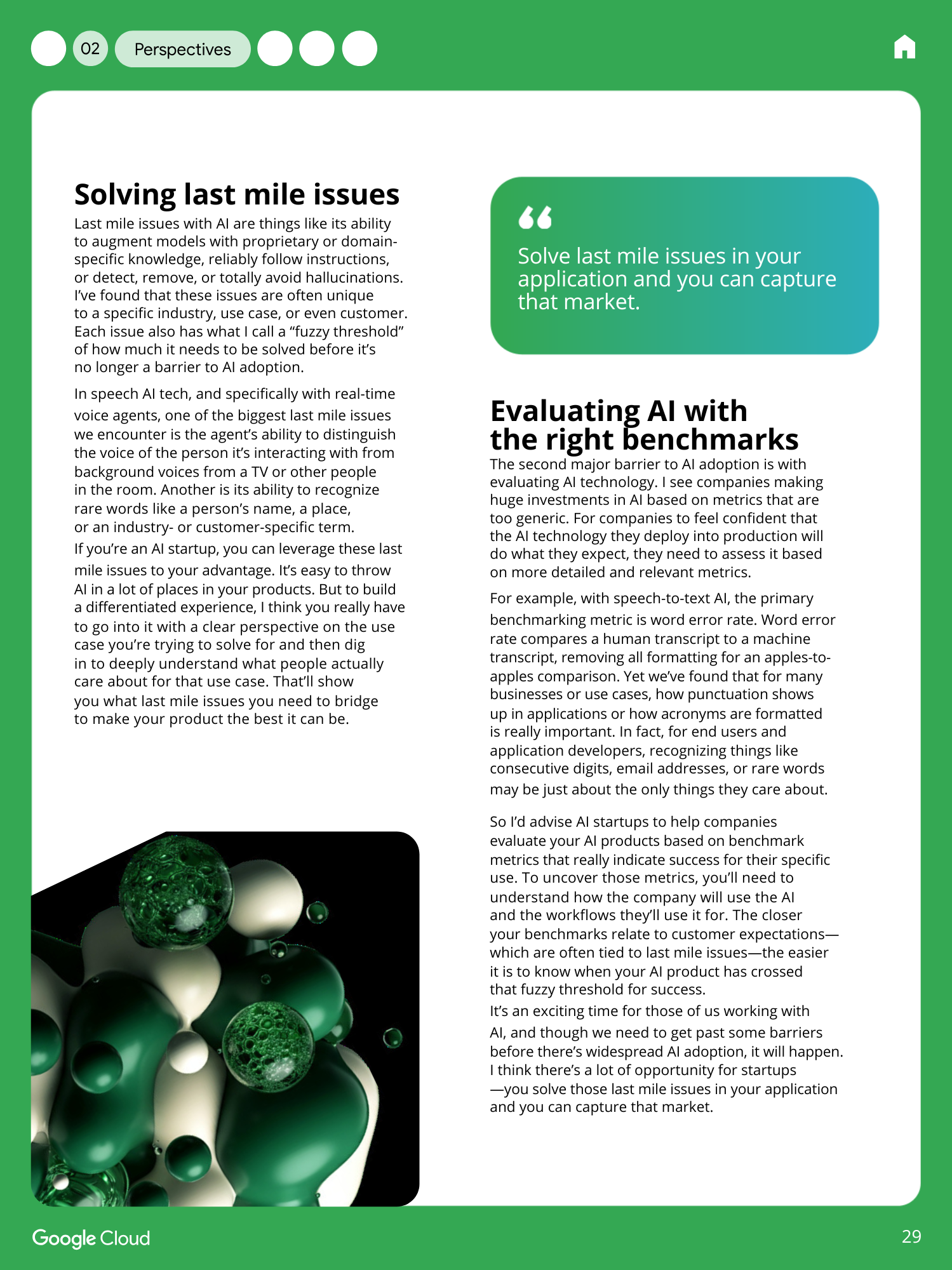
Bridge the gap of last mile issues
1. Differentiate your startup by addressing last mile issues and using nuanced benchmarks for AI performance
I’m excited about how real-time, AI-driven agents will evolve. We’ll see multimodal agents that will include visual and audio in their interactions. For example, rather than calling a plumber for help with a leaky pipe, you’ll open an app, show where the pipe is leaking, and the agent will talk you through fixing it in real time.
The timeline for widespread agent adoption and of AI in general will be longer than people think. I say this for a few reasons:
- We clearly have to address a ton of last mile issues that aren’t obvious until you’re deep into production. The user experience with AI still has some clunkiness that’ll need to be smoothed out—things like interruptions and delays in responses that make you realize you’re not talking to a human.
- I also think that companies using and implementing AI tech will need to change how they evaluate it to get the results they expect. The silver lining is that these same last mile issues and difficulties evaluating AI tech offer startups the chance to differentiate themselves.
2. Solving last mile issues
Last mile issues with AI are things like its ability to augment models with proprietary or domainspecific knowledge, reliably follow instructions, or detect, remove, or totally avoid hallucinations. I’ve found that these issues are often unique to a specific industry, use case, or even customer. Each issue also has what I call a “fuzzy threshold” of how much it needs to be solved before it’s no longer a barrier to AI adoption.
In speech AI tech, and specifically with real-time voice agents, one of the biggest last mile issues we encounter is the agent’s ability to distinguish the voice of the person it’s interacting with from background voices from a TV or other people in the room. Another is its ability to recognize rare words like a person’s name, a place, or an industry or customer-specific term.
If you’re an AI startup, you can leverage these last mile issues to your advantage. It’s easy to throw AI in a lot of places in your products. But to build a differentiated experience, I think you really have to go into it with a clear perspective on the use case you’re trying to solve for and then dig in to deeply understand what people actually care about for that use case. That’ll show you what last mile issues you need to bridge to make your product the best it can be.
Evaluating AI with the right benchmarks
The second major barrier to AI adoption is with evaluating AI technology. I see companies making huge investments in AI based on metrics that are too generic. For companies to feel confident that the AI technology they deploy into production will do what they expect, they need to assess it based on more detailed and relevant metrics.
For example, with speech-to-text AI, the primary benchmarking metric is word error rate. Word error rate compares a human transcript to a machine transcript, removing all formatting for an apples-toapples comparison. Yet we’ve found that for many businesses or use cases, how punctuation shows up in applications or how acronyms are formatted is really important. In fact, for end users and application developers, recognizing things like consecutive digits, email addresses, or rare words may be just about the only things they care about.
So I’d advise AI startups to help companies evaluate your AI products based on benchmark metrics that really indicate success for their specific use. To uncover those metrics, you’ll need to understand how the company will use the AI and the workflows they’ll use it for. The closer your benchmarks relate to customer expectations— which are often tied to last mile issues—the easier it is to know when your AI product has crossed that fuzzy threshold for success.
It’s an exciting time for those of us working with AI, and though we need to get past some barriers before there’s widespread AI adoption, it will happen. I think there’s a lot of opportunity for startups —you solve those last mile issues in your application and you can capture that market.
Read the full Google Cloud Future of AI: Perspectives for Startups report here.
Lorem ipsum dolor sit amet, consectetur adipiscing elit, sed do eiusmod tempor incididunt ut labore et dolore magna aliqua. Ut enim ad minim veniam, quis nostrud exercitation ullamco laboris nisi ut aliquip ex ea commodo consequat. Duis aute irure dolor in reprehenderit in voluptate velit esse cillum dolore eu fugiat nulla pariatur.