Releasing our new v9 transcription model - 11% better accuracy
Today, we’re excited to release our most accurate Speech Recognition model to date - version 9 (v9). This updated model delivers increased performance across many metrics on a wide range of audio types.



Today, we're excited to announce our new v9 transcription model. The v9 model marks one of our biggest improvements to date and shows increased performance across the board on many audio types compared to our v8 model.
The v9 model also provides the foundation for our v10 model, which our AI research team is already working on for release in early 2023. Building on the improvements of v9, the v10 model is expected to provide a radical improvement in speech recognition accuracy.
Overall Improvements
Word Error Rate, or WER, is the primary quantitative metric by which the performance of an automatic transcription model is measured. WER effectively reports the percentage of words in a transcription that differ from what was actually said. Our new v9 model shows significant improvements across a range of different audio types, as seen in the chart below, with a more than 11% improvement on average.
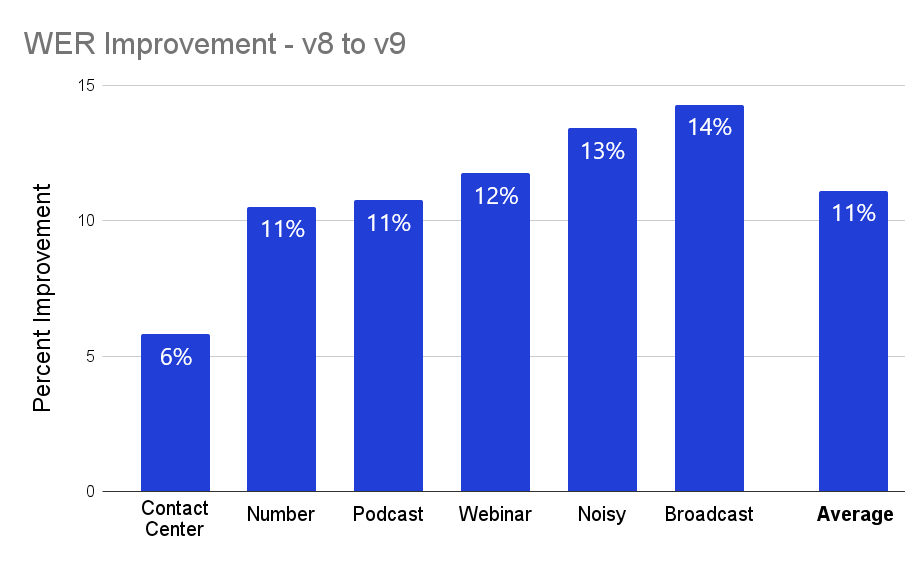
Let's take a look at a specific example, using a clip (starting at about 8:30) from this Lex Fridman podcast with Yann LeCun. The transcripts generated by both the v8 and v9 model can be seen below with differences in the transcripts highlighted. The v9 model produces a more accurate, readable transcript that more faithfully captures the semantics of the conversation as implied by the speakers' tones.
Lex Fridman Podcast
0:00
/1:27
1×
v8 Transcript
v9 Transcript
And so, obviously, we're missing something, right? And it's quite obvious for a lot of people that the immediate response you get from many people is, well, humans use their background knowledge to learn faster, and they are right. Now, how was that background knowledge acquired? And that's the big question. So now you have to ask, how do babies in the first few months of life learn how the world works? Mostly by observation, because they can hardly act in the world, and they learn an enormous amount of background knowledge about the world that may be the basis of what we call common sense. This type of learning is not learning a task. It's not being reinforced for anything. It's just observing the world and figuring out how it works, building world models, learning world models. How do we do this, and how do we reproduce this in machines? So, self supervised learning is one instance or one attempt at trying to reproduce this kind of learning. Okay, so you're looking at just observations. So not even the interacting part of a child, it's just sitting there, watching mom and dad walk around, pick up stuff, all of that. That's that's what you mean by background knowledge. Perhaps not even watching mom and dad just, you know, watching the world go by, just having eyes open or having eyes closed or the very act of opening and closing eyes that the world appears and disappears. All that basic information.
Proper Nouns
In addition to standard overall WER, the new v9 model shows marked improvements with respect to proper nouns. This means that words like company, product, and human names will be more accurately transcribed. In the chart below, we can see the relative performance increase of v9 over v8 for various types of audio, with a nearly 15% improvement on average.
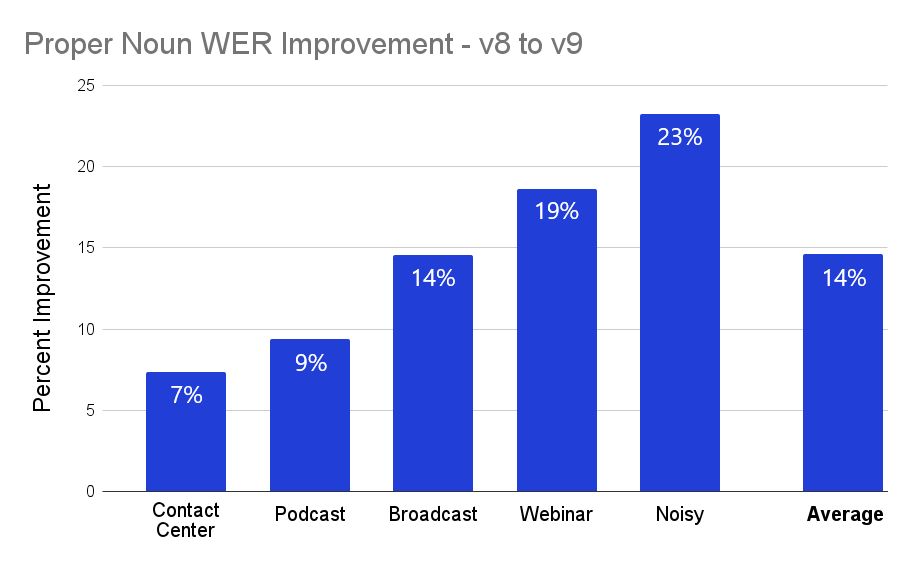
Beyond WER, we can look at a few examples of transcripts to see that v9 outperforms v8 when it comes to proper nouns:
v8 Transcript
v9 Transcript
- … just make another Shrek if you want to make another Shrek for that …
- … i’m calling on behalf of Stanfield Real Estate Sotheby's international …
- … they’re more like text editors that have a lot of add ons but PyCharm …
Improved transcription quality
Quantitative metrics like WER provide valuable insight into model performance, but they don't give us the full picture. Other considerations like punctuation, automatic formatting, and more impact the readability of transcripts. At the end of the day, automatic transcription exists to make the lives of humans easier, so it's important to consider human evaluation as well.
To show that the increased performance of v9 runs deeper than quantitative metrics, we ran blind experiments in which a reader was asked to choose which (if either) of two transcripts was superior - one from our v8 model and one from our v9 model. We found that in the majority of cases, participants preferred v9's transcripts over v8's, and almost nobody preferred v8's.

Further, when asked to evaluate performance considering only proper nouns, we found similar results:

Powered by new AI research
Our dedicated in-house team of AI researchers is constantly designing new models to push forward the state of the art in both automatic transcription and audio intelligence. These ever-evolving models ensure that our customers always receive the best possible results that cutting-edge research affords. The new v9 model features an improved audio encoder architecture — an Efficient Conformer — which contributes to its elevated performance.
Model architecture is only one half of the equation — the best models are also necessarily trained on a lot of high-quality data. To get the most out of v9's architectural improvements, our AI research team curated tens of thousands of hours of new, specialized audio data to supplement the model training process.
What this means
The new v9 transcription model is currently deployed and in use by our customers. This means that customers will see improved performance with no changes required on their ends. The new model will automatically be used for all transcriptions going forward, with no need to upgrade for special access.
While our customers enjoy the elevated performance of the v9 model, our AI research team is already hard at work on our v10 model, which is slated to launch in early 2023. Building upon v9, the v10 model is expected to radically improve the state of the art in speech recognition.
Our customers will continue to use our models to build high-ROI features that give them a competitive edge, like Veed's automatic captioning system, Spotify's targeted advertising platform, or CallRail's call tracking and conversational intelligence platform.
Try our new v9 transcription model through your browser using the AssemblyAI Playground. Alternatively, sign up for a free API token to test it out through our API, or schedule a time with our AI experts to learn more.
Lorem ipsum dolor sit amet, consectetur adipiscing elit, sed do eiusmod tempor incididunt ut labore et dolore magna aliqua. Ut enim ad minim veniam, quis nostrud exercitation ullamco laboris nisi ut aliquip ex ea commodo consequat. Duis aute irure dolor in reprehenderit in voluptate velit esse cillum dolore eu fugiat nulla pariatur.