AI Models for Smart Media Monitoring
Learn how AI Models help facilitate smarter media monitoring.

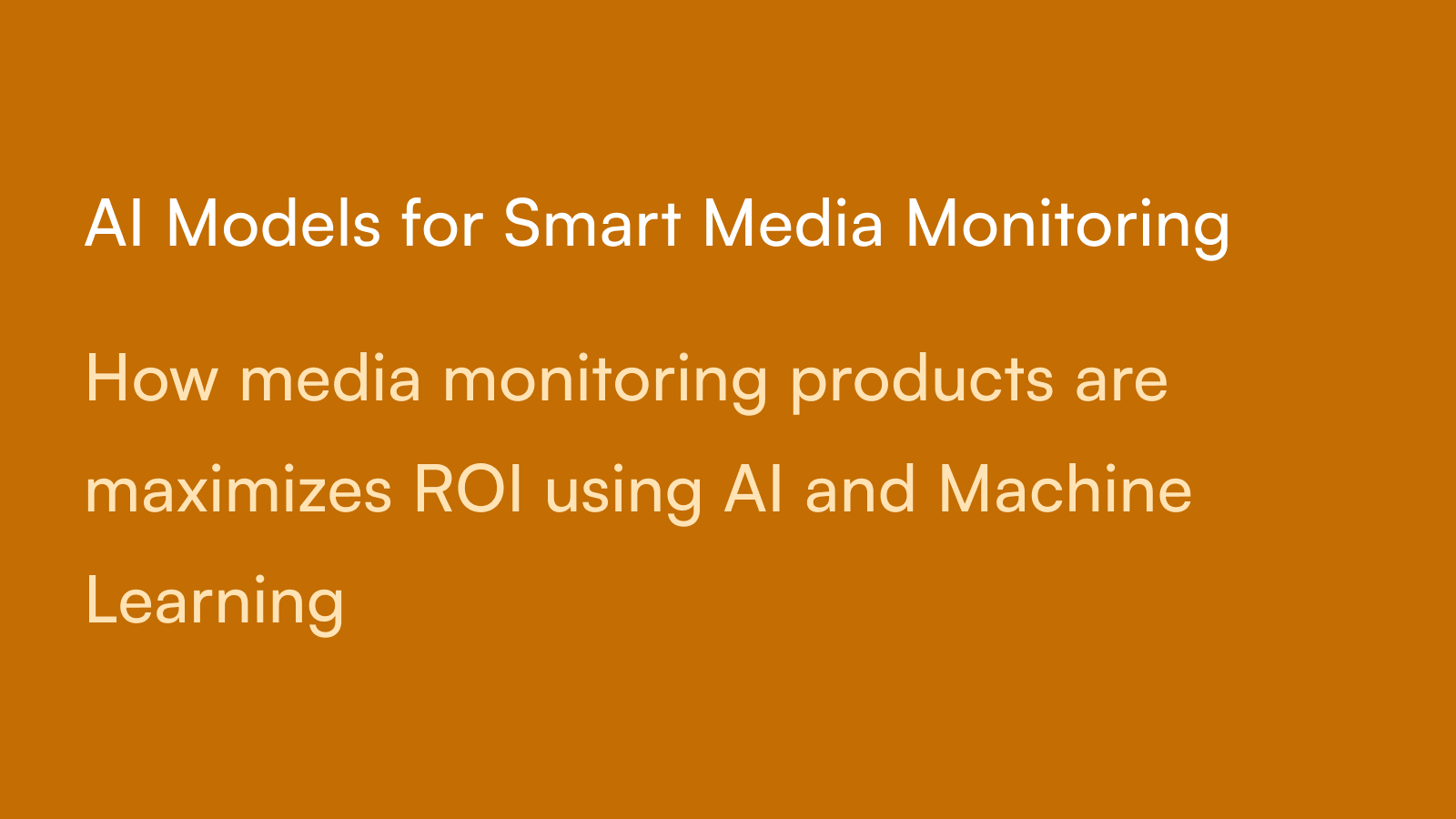

Brands need to know who’s talking about them, how often, and in what context. But with over 720,000 hours of video uploaded per day on YouTube alone, how can they effectively sift through the noise to pinpoint only what they need to know?
Speech-to-Text transcription is a great starting point for effective media monitoring. But in today’s fast paced, media saturated world, there is only so much you can do with just a static transcription text.
In order to build a media monitoring product that truly maximizes ROI, you also need to apply AI models to understand context as well. Then, all of this data can be aggregated so brands can more effectively monitor mentions and perform intelligent analytics.
At AssemblyAI, we refer to these high-value NLP and NLU tools as Audio Intelligence.
Audio Intelligence APIs can help in two distinct ways:
- By offering accurate proper noun recognition to ensure all brand name mentions are captured.
- By understanding the context in which a brand is mentioned.
Let’s look at how this works in more detail.
Looking for an AI Model?
Learn why developers choose AssemblyAI's State-of-the-Art AI models.
Accurate Proper Noun Recognition with Speech-to-Text APIs
AssemblyAI’s Automatic Punctuation and Casing Model is trained on text with billions of words, making our proper noun ASR model the most accurate on the market today.
Because our model is trained on such an abundance of data, our Speech-to-Text API and ASR models can accurately predict even the most obscure proper nouns. In addition, our Word Boost feature lets companies add custom vocabulary words or custom casings to increase transcription accuracy further.
Why is this important?
With so much data to filter, you need to ensure that (A) companies minimize the chance that the wrong brand name is detected and (B) maximize the chance that all mentions of a brand name are accounted for.
Audio Intelligence and Smart Media Monitoring
Built using cutting edge Machine Learning, Deep Learning, and NLP research, Audio Intelligence APIs let customers quickly build high ROI features and applications on top of their audio data–helping brands move beyond basic media monitoring.
For companies looking to develop intelligent media monitoring products, Audio Intelligence tools can help give insight into:
- The volume of brand mentions across online platforms and across set time periods.
- The share of brand mentions by platform compared to other brands.
- The sentiment associated with a brand mention.
- The context associated with a brand mention.
- Detailed analysis for each of the above.
Below, we’ll examine the top four Audio Intelligence tools that help us accomplish this Smart Media Monitoring: Topic Detection, Entity Detection, Content Moderation, and Sentiment Analysis.
For each one, we’ll look at what they are, how they work, and how they maximize ROI.
Topic Detection
By leveraging large NLP models, Topic Detection APIs can understand the context of what is spoken in an audio or video file or in a text and then use this context to accurately predict the topics that are discussed.
By using Topic Detection, you can tell if a brand name is being mentioned more often in relation to topics a brand does, or does not, want it to be associated with. Does a brand want to be always associated with football
or parks
? Topic Detection can help ensure this is happening.
Topics are labeled according to the standardized IAB Taxonomy, a compilation of 698 common topics. These include:
For example, the AssemblyAI Topic Detection API was able to determine that this short transcription was about baseball
simply by referencing an MLB pitcher:
In my mind, I was basically done with Robbie Ray. He had shown flashes in the past, particularly with the strike. It was just too inefficient walk too many guys and got hit too hard too.
Entity Detection
Entity Detection (A) identifies and (B) categorizes key information in a transcription text. For example, French
is an entity that is classified as a language
.
By using Entity Detection, you can determine if a brand name is mentioned in the context of other organizations, like a national news site or a competitor name, specific events, specific locations, and more.
Entity Detection APIs can classify the following 25 entities in a text:
For example, in the following transcription text, the AssemblyAI Entity Detection API was able to label person_name
, phone_number
, and occupation
as entities mentioned.
John Bishop is a photographer from Chicago, Illinois. His phone number is 111-333-1111.
Content Moderation
Another way to approach Smart Media Monitoring is through Content Moderation.
Content Moderation APIs help spot red flags associated with brand mentions – does a brand name appear next to content that includes sensitive_social_issues
, profanity
, or negative news
? Is there some action to take if it is?
Content Moderation gives you this insight.
Content Moderation topics that can be flagged include:
For example, in a sample Ted Talk audio file, the AssemblyAI Content Moderation API detected health_issues
in the following text:
Yes, that's it. Why does that happen? By calling off the Hunt, your brain can stop persevering on the ugly sister, giving the correct set of neurons a chance to be activated. Tip of the tongue, especially blocking on a person's name, is totally normal. 25 year olds can experience several tip of the tongues a week, but young people don't sweat them, in part because old age, memory loss, and Alzheimer's are nowhere on their radars.
Sentiment Analysis
Tracking social sentiment, or the mood of the conversation, is another valuable component of Smart Media Monitoring. Companies looking to accomplish this do so through an Audio Intelligence tool called Sentiment Analysis.
Sentiment Analysis APIs detect positive
, negative
, or neutral
sentiments in a text or speech segment. For example, the AssemblyAI Sentiment Analysis API identified the following speech segment:
Ted Talks are recorded live at Ted Conference.
as NEUTRAL
.
And
His episode features psychologist and happiness expert Dan Gilbert.
as POSITIVE
.
Sentiment Analysis is a useful tool for determining attitudes toward products, brands, events, and more. Is a brand name mentioned more in content that is positive or negative? Are certain products mentioned more in content that is positive or negative? By collecting this data, a brand can be better equipped to take informed action.
Smarter Media Monitoring
Today’s brands must work strategically to stay competitive. One intelligent approach is to leverage the vast amounts of data available online with the help of ASR and Audio Intelligence.
By using Audio Intelligence tools like Content Moderation, Entity Detection, Topic Detection, and Sentiment Analysis, Smart Media Monitoring solutions can help companies gain a clearer picture of what’s being said about their brand and in what context. Then, they can analyze this data to make more informed brand decisions and increase ROI when building out media monitoring products.
Ready for smarter media monitoring?
Chat with one of our team members to learn why hundreds of businesses, including dozens of Fortune 500s, process millions of audio files every day with AssemblyAI's platform of APIs for State-of-the-Art AI Models.
Lorem ipsum dolor sit amet, consectetur adipiscing elit, sed do eiusmod tempor incididunt ut labore et dolore magna aliqua. Ut enim ad minim veniam, quis nostrud exercitation ullamco laboris nisi ut aliquip ex ea commodo consequat. Duis aute irure dolor in reprehenderit in voluptate velit esse cillum dolore eu fugiat nulla pariatur.