Build or buy? What industry leaders are choosing
We’re tackling the industry’s biggest question with key learnings from our 2024 Insights Report. Learn how leaders are addressing integration challenges and whether they’re building in house, on open-source models, or with AI providers.



In 2024, the benefits of integrating AI into business processes and products is clear—but the route to that integration is not quite as straightforward. Despite an undeniable eagerness to integrate, organizations are forced to answer one critical question before they can—build in-house, on open-source tools, or with AI providers?
Each path has a unique set of benefits and drawbacks, and it’s essential to understand what those are in order to make the best decision for your business and goals. A wrong route is no small setback and can result in major delays, resourcing constraints, and financial burdens.
We surveyed more than 200 tech founders to find out what the primary barriers to AI integration are and what their teams are doing to work around them.
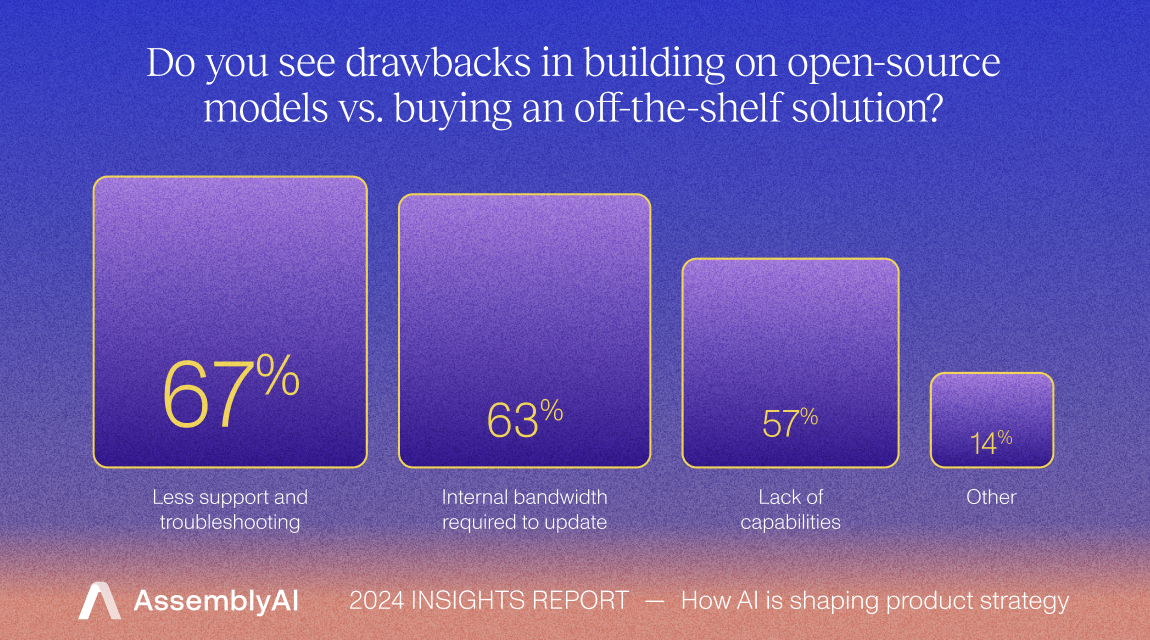
Barriers to AI integration
Despite widespread interest in AI, many organizations struggle to implement it effectively due to 5 primary barriers.
Technical Complexity
Building AI systems requires advanced expertise in machine learning, data engineering, and infrastructure management. For organizations without these skill sets, the learning curve can be overwhelming. Our report indicated that 49% of leaders cited the learning curve as a top challenge when considering open-source or in-house options.
Team Bandwidth
On average, 52% of our respondents reported insufficient team capacity as a major barrier. Developing AI solutions in-house requires substantial time commitments from initial integration to ongoing operations and updates. Many organizations find their teams stretched thin, making it difficult to dedicate the appropriate amount of energy to the rigorous demands of AI development and deployment.
Cost
AI projects demand substantial investment—not just in the technology but also in the talent. Integrating AI requires top-tier talent, plus additional resources allocated for infrastructure, ongoing model maintenance, security best practices and more. Many companies underestimate the costs of building or scaling AI systems, leading to budget overruns and unmet expectations.
Speed of iteration
Moving quickly means always having dedicated hands on deck. When engineering and development teams are responsible for both developing and maintaining custom AI along with designing and building the customer-facing tools and features, the question of whether or not they’ll be able to move fast enough becomes a big one.
Data security, privacy, and compliance
Organizations must ensure that sensitive data, such as financial or medical information, is properly secured and stored. This includes implementing encryption protocols and deciding whether customer data will be used for training models. Legal and regulatory concerns, such as GDPR or HIPAA, must also be addressed. Businesses need a clear plan for meeting these requirements and maintaining compliance with relevant laws. Ongoing monitoring and support are necessary to adapt to changing regulations and safeguard against security risks.

In-House, Open Source, or AI Provider?
Building from the ground up can be tempting—it lends itself to pure control and customization—however, as many industry leaders are learning, it’s not a temptation that comes without complexity. Businesses are limited to their own capabilities and are quite often caught off guard by the unforeseen complications, questions, and costs that accompany in-house and open-source builds.
Building In-House
While this DIY approach can yield expert solutions tailored to hyper-specific needs, it is resource intensive and risky.
- Pros:
- Full control over the AI model and data, but only if your expertise allows
- Custom solutions optimized for niche use cases
- Cons:
- Requires significant investment in hiring AI talent and infrastructure
- Long development timelines and unforeseen deployment delays
Open-source
Open-source frameworks like TensorFlow and PyTorch offer a middle ground, enabling teams to build AI systems without starting from scratch. These tools are free and widely supported by developer communities.
- Pros:
- Cost-effective and flexible
- Access to pre-built models and shared innovations
- Cons:
- Limited support for scaling beyond prototypes
- Ongoing maintenance and expertise are still required
- Less customization options
Partnering with AI Providers
AI providers deliver cutting-edge yet ready-made solutions that simplify implementation. These platforms often include APIs for specific tasks like speech recognition, image analysis, or natural language processing and allow businesses to work alongside the best experts in the business.
- Pros:
- Faster time to market thanks to reduced technical barriers and deployment timelines
- Pre-trained models with high accuracy
- Dedicated support teams
- Cons:
- Reliance on a third party
- Ongoing subscription costs

Asking the right questions
The question of build or buy can be a daunting one. With a sea of moving parts and an endless list of goals, it can be overwhelming for teams to clearly define the best path forward. For anyone considering the pros and cons of various AI integration options, having answers to the right questions—and knowing what those questions are—is paramount.
- How will you ensure the model achieves and maintains continuous, state-of-the-art accuracy?
- How will you manage competing engineering and development priorities?
- How will you source diverse, robust training data?
- How will the model handle custom vocabulary needs?
- Do you have the internal expertise to build or will you need to hire additional experts?
- Do you have a thorough understanding of AI and speech recognition best practices?
- How will you handle the ongoing maintenance of the model?
- How will you quickly handle mission-critical support requests?
- Do you have a clear understanding of the startup and ongoing costs of building in-house versus partnering with a third party?
- How will you ensure data security and compliance?
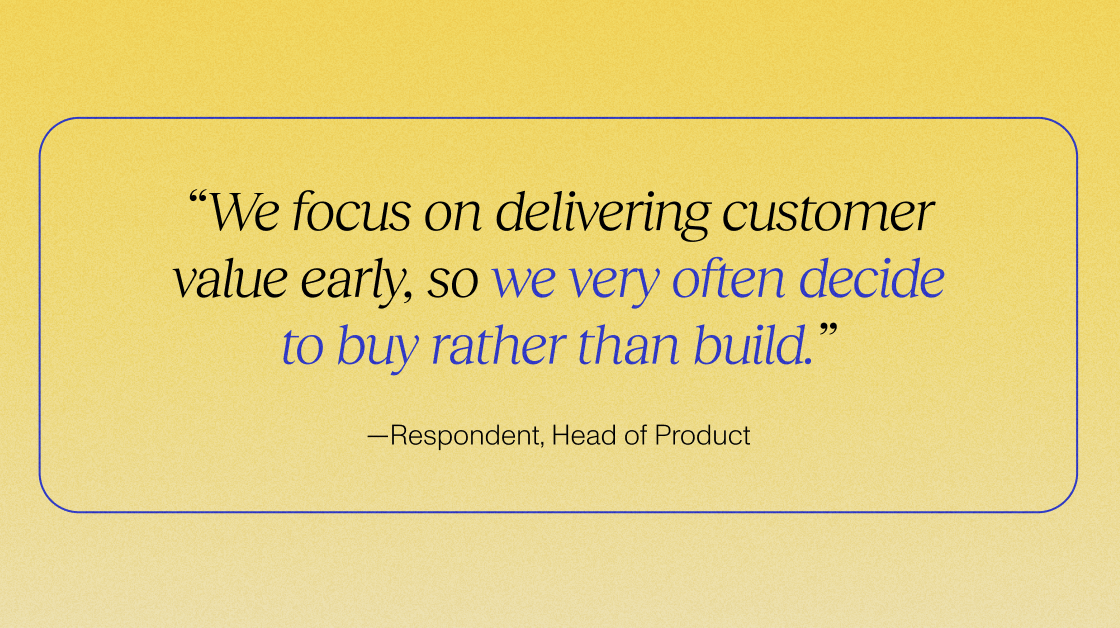
Key takeaways
AI holds transformative potential, but its integration comes with challenges that demand careful consideration. Whether you choose to build in-house, leverage open-source tools, or partner with an AI provider, the right approach depends on your organization’s unique goals, resources, and expertise.
Despite a significant learning curve and the need for staffed AI experts, industry leaders are no less eager to integrate. What our insights showed as the primary workaround to these challenges, is a strategic partnership with AI providers that deliver cutting-edge capabilities that don’t require heavy lifting from your team. These partnerships not only free up internal business resources, but they allow teams to think bigger and move faster than ever before.
Get the full picture of what industry leaders are doing in the age of AI and how they approached the question of build or buy, in our 2024 Insights Report.
Click here to access the full research report:
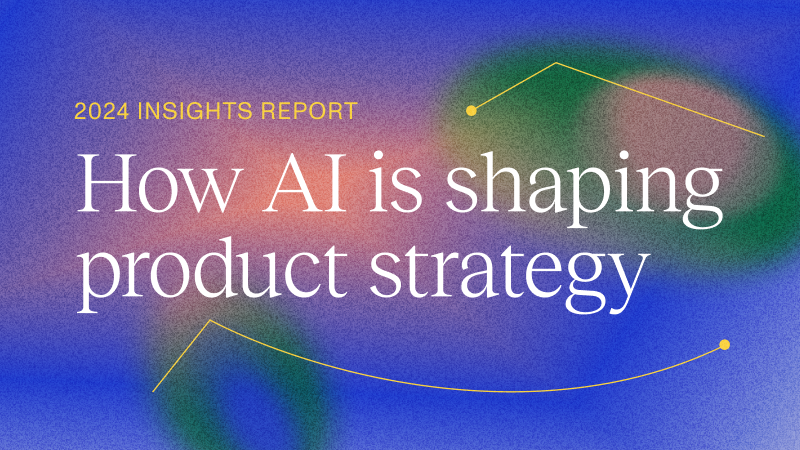
Lorem ipsum dolor sit amet, consectetur adipiscing elit, sed do eiusmod tempor incididunt ut labore et dolore magna aliqua. Ut enim ad minim veniam, quis nostrud exercitation ullamco laboris nisi ut aliquip ex ea commodo consequat. Duis aute irure dolor in reprehenderit in voluptate velit esse cillum dolore eu fugiat nulla pariatur.