Review - Text-Free Prosody-Aware Generative Spoken Language Modeling
In this week's Deep Learning Paper Review, we look at the following paper: Text-Free Prosody-Aware Generative Spoken Language Modeling.



In this week's Deep Learning Paper Review, we look at the following paper: Text-Free Prosody-Aware Generative Spoken Language Modeling.
What's Exciting about this Paper
This paper is the first published work to include prosody as a feature for generative spoken language modeling. There has been previous work on generative spoken language modeling without prosody information, and previous work on discriminative speech classification tasks using prosody features, but this work is the first to pull them together.
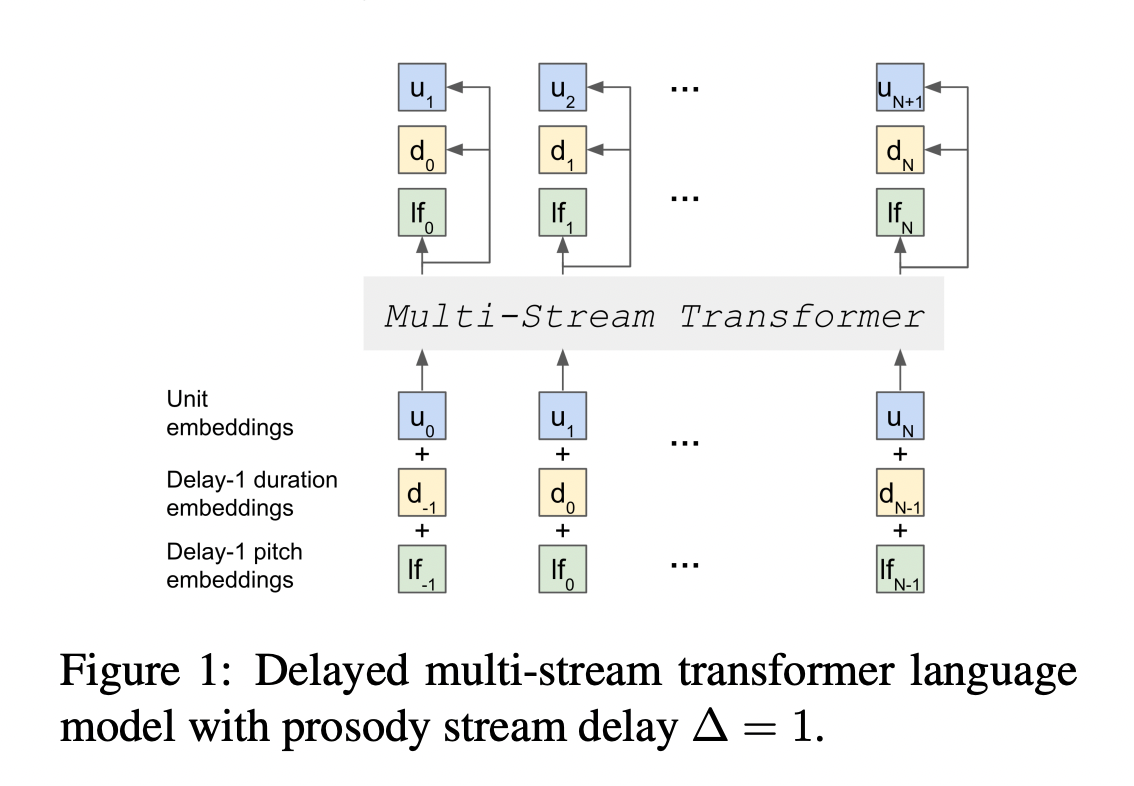
The fundamental application here is to take speech input as a prompt, and generate coherent and consistent waveform speech output. The more "macro" purpose of this research is to improve speech-based dialogue systems.
The driving hypothesis is that text, the de facto intermediate representation between speech inputs and any NLP-style analyses, is suboptimal: even when adequately available, text is a lossy medium for capturing speech.
By incorporating prosody, and directly modeling in the spoken language domain, without cascading through text, this work attempts a more optimal representation.
The features the authors leverage are self-supervised, discovered acoustic units representing phonetic content; and quantized, speaker-mean normalized, log F0
bins together with unit durations representing prosody. They model these three input streams jointly with a transformer language model, and use a HiFi-GAN vocoder to convert the LM outputs into a waveform. They devise new metrics to complement existing ones as they measure the accuracy, consistency, and expressiveness of generated speech.
The Paper's Key Findings
They find the prosodic input features universally improve content modeling as well as prosody modeling. There are several other findings, but that’s the punchline: prosody helps!
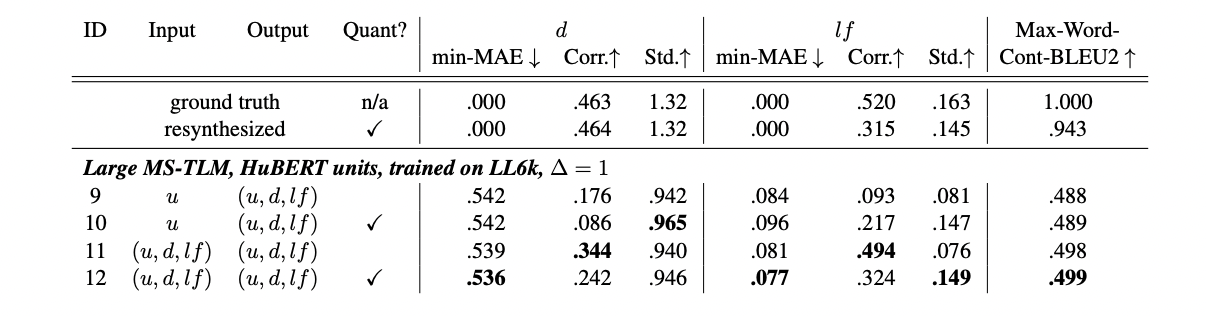
Our Takeaways
This work is very very cool, but we would emphasize that it is a novel, exploratory research direction. When listening to the samples released with the paper, we found them hard to follow—which we think would have made the human evaluation task exceedingly difficult.
For us, this work has clarified the destination but left the path uncertain: it seems inevitable that spoken language modeling will abandon the cascade and go end-to-end, but we certainly aren’t there yet!
Lorem ipsum dolor sit amet, consectetur adipiscing elit, sed do eiusmod tempor incididunt ut labore et dolore magna aliqua. Ut enim ad minim veniam, quis nostrud exercitation ullamco laboris nisi ut aliquip ex ea commodo consequat. Duis aute irure dolor in reprehenderit in voluptate velit esse cillum dolore eu fugiat nulla pariatur.